Projects
New remote non-invasive monitoring solutions for ensuring the health of mothers and babies before and after birth
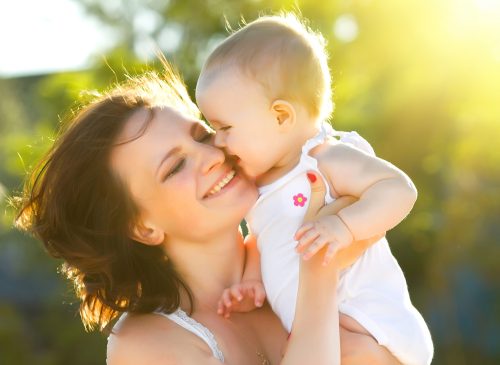
36 months (01/01/2023 – 31/12/2025)
25M€ total, 1.5M€ University of Turku
Every year more than 140 million new babies are born, of which 15 million are born too early. Complications during birth account for 40 % of perinatal and maternal deaths (over 287 000 worldwide2). In Europe, about 5 million babies are born early, and of these pre-term babies, about 10 % cause a severe cost burden to healthcare – not only due to immediate short-term costs of intensive neonatal care but most importantly, long-term adverse effects life-long complications. Many preterm births could be prevented with careful pregnancy monitoring and early prediction and detection of risks and complications. Many solutions focus on in-hospital settings, but in Newlife we see the need for medically reliable; cost-effective wearable device to be on the market for pregnancy self-monitoring that could enable closer observation, provide reassurance to concerned expectant mothers, and better identify high-risk pregnancies patients.
The NEWLIFE project will answer to the ongoing digital transformation in healthcare from the on-site hospital environment to remote healthcare in the home environment, by demonstrating digital and technological solutions serving both parents and new-borns, securing life in its most vulnerable phase. The target is to follow the P4 approach from medicine (i.e., predictive, preventive, personalized and participatory)4 to develop new solutions, both hardware and software, for accurate measurements and acquisition of vital data, focusing on non-invasive, comfortable, and wearable solutions that are easy to use and sustainable.
The focus is to build solutions for screening and early detection of pregnancy risk factors, such as hypertension, diabetes, pre-term labour or pre-eclampsia or risk of fetal death, where the healthcare professionals are always integrated into the solution which will enable pre-emptive support and treatment for mothers and their unborn babies. The identified complications might then lead to more detailed, continuous, and personalised monitoring during pregnancy, enabled by novel wearable solutions to be used both at home and hospital care. After birth, babies with identified risks or complications need constant monitoring both in the neonatal hospital care and after discharge, to co-care in hospital environment or at home. Ideally, the same technology and solutions should be available and used both in hospital and home environment to facilitate the move to remote monitoring and ease the data integration.
Bringing together universities, companies and hospitals to tackle the design and the early-phase validation of digital biomarkers
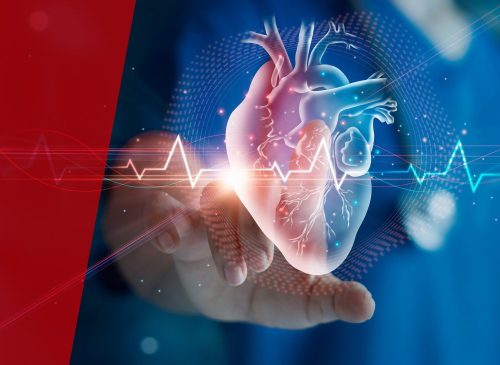
INSIDE-HEART brings together universities, companies and hospitals from countries (Italy, Finland, France, Israel, Netherlands, Spain, and Sweden) with the main scope of establishing a multi-disciplinary network to tackle the design and the early-phase validation of digital biomarkers, specifically targeting the diagnosis of supraventricular arrhythmias (SVAs) and their associated potential for adverse risk assessment, via the joint combination of signal processing, artificial intelligence and non-clinical devices. This will be achieved by performing excellent research through a unique doctoral training “without walls” among field-expert academic, industrial and clinical entities.
The composite nature of the INSIDE-HEART network ensures a highly qualified training and research infrastructure for the specific goal, which aims to generate a new profile of researcher with multi-sectoral expertise able to fill the existing gap, i.e., the absence of digital biomarkers for SVAs reliably estimated with non-clinical devices, taking into account basic research, clinical needs and business interests. Research and training are designed to consider relevant aspects such as public concern of private data management, gender and ethics related to SVAs, all according to Responsible Research & Innovation principles and Open Science practices.
All activities in INSIDE-HEART are designed to pursue innovation in three domains: i) Educational domain – by implementing a new multi-sectoral paradigm of PhD training to shape modern professional researchers with cross-competencies in the field, and able to accelerate the translation from basic science to market and clinics; ii) Basic science domain – by producing new knowledge about digital biomarkers by means of a multi-sectoral approach to explore the complex aspects related to SVAs; and iii) Technological domain – by developing new data-driven and model-based methodologies to compute digital biomarkers and support the clinical decision.
Privacy compliant health data as a service for AI development
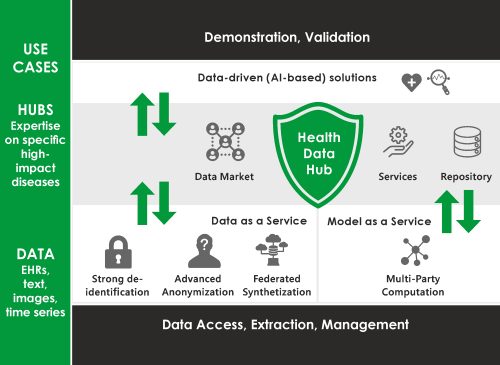
36 months (01/10/2023 – 31/09/2026)
6.6M€ total, 929000€ University of Turku
The overall aim of PHASE IV AI is to increase the availability of real-world evidence by providing privacy compliant health data as a service. Artificial intelligence (AI) enables data-driven innovations in health care. AI systems, which process vast amounts of data quickly and in detail, show promise both as a tool for preventive health care and clinical decision-making. However, the distributed storage and limited access to health data form a barrier to innovation, as developing trustworthy AI systems requires large datasets for training and validation.
Furthermore, the availability of anonymous datasets would increase the adoption of AI-powered tools by supporting health technology assessments and education.
Secure, privacy compliant data utilization is key for unlocking the full potential of AI and data analytics. Companies developing AI solutions would benefit from synthetic microdata for early-stage development, provided on-demand and with privacy guarantees. For researchers and clinicians interested in aggregate data or modelling, multi-party computation allows deriving insights from the distributed real-world data. In this way, providing synthetic data and multi-party computation as a service will boost data-driven innovation without compromising the privacy of data subjects.
Machine learning for secure multi-hospital data integration
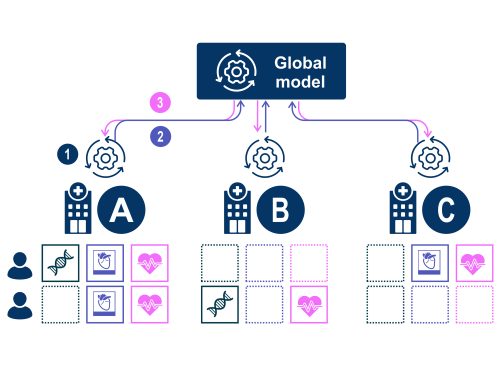
36 months (01/01/2024 – 31/12/2026)
453000€
This project aims to improve healthcare by harnessing the potential of health data and artificial intelligence. We develop machine learning based methods that allow secure and private analysis of health information from multiple sources, such as medical records, wearable devices, and medical images. This will support doctors in making well-informed decisions for preventive care, early diagnosis, and personalized medicine.
Our approach focuses on three key aspects: creating efficient algorithms to handle diverse health data, ensuring security and privacy through advanced techniques, and testing our methods on real-world cases, specifically cardiovascular diseases.
Speckle Technology and Digital Biomarkers of Microvascular Function for Monitoring Cardiovascular Diseases
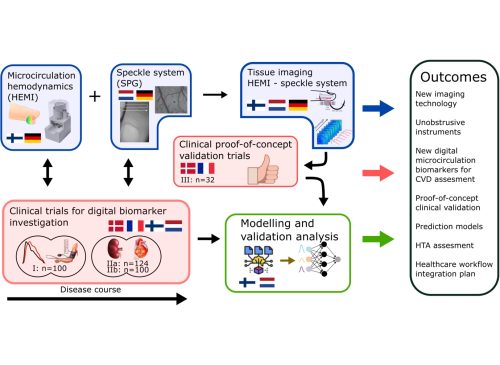
48 months (01/10/2023 – 30/09/2027)
3 979 007€ total, 1 336 149€ University of Turku
STIMULUS project develops new non-invasive, convenient, low-cost and easy-to-use imaging technology that can measure new digital biomarkers from microcirculation for early detection of cardiovascular diseases. The novel technology will enable earlier detection of heart failure and chronic kidney disease, which will substantially decrease cardiovascular events in the European population.
For both heart failure and chronic kidney disease patients, it is important to diagnose microvascular dysfunction and monitor the deterioration of microvascular function in early phases of the disease progress. Our proposed technology HEMI-speckle system is an integrated solution of two existing prototypes, namely, the multi-spectral optical sensory system and the speckle plethysmography (SPG) system.
Synthetic Health Data Testbed
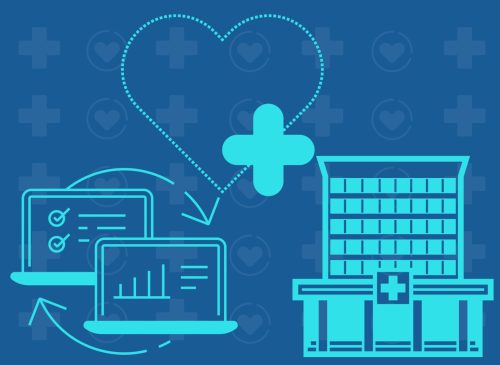
24 months (01/04/2023 – 31/03/2025)
495 000€ total, 190 703€ University of Turku
SYNDATE answers the companies’ needs for data-driven product development. In this project, we will create a testbed that offers access to synthetic data that looks and behaves like realworld data. By doing this, we provide a partial solution to the bottlenecks of the secondary use of health and social data. We will use advanced modeling techniques that ensure the synthetic data closely matches the properties of the real-world data. The selected techniques include settings to preserve privacy, which makes it possible to fulfill strict data protection criteria. This way, the developers and innovators may gain access to synthetic datasets without the need for heavy regulatory processes.
The testbed with synthetic data allows developing new health-data based solutions, for example using artificial intelligence, much faster than previously possible. The product development in companies becomes faster and more efficient while decreasing the development cost. The developed service also benefits health care providers and patients via new improved technological solutions.
The testbed is a service developed for companies’ and research organizations. Not only will it strengthen the local health ecosystem, Health Campus Turku, but also support the RDI on national level. The core aim of SYNDATE is to facilitate industry-driven innovation in the health sector. The testbed, created through co-creation, also opens a new collaboration channel for research organizations and companies. What’s more, the project builds upon and further develops the synthetic data competencies in the Turku area.
Remote Monitoring in Health and Sports
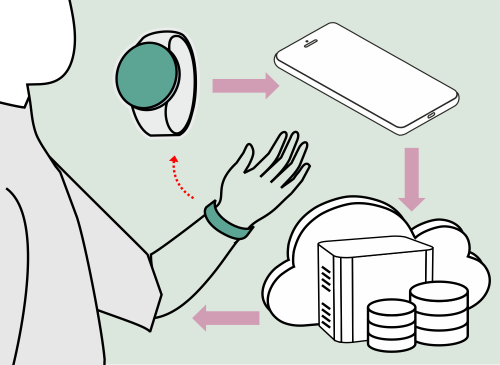
36 months (01/01/2023 – 31/12/2025)
€1.1M University of Turku
Wearable health monitoring systems provide a big promise of allowing individuals to closely monitor changes in their vital signs and provide feedback to regain or maintain an optimal health status. The RM4Health project will accelerate innovation in electronic wearable devices. RM4HEALTH will focus on the development of open technology platforms for vital sign monitoring for these emerging fields to help them bridge ‘the Valley of Death’ in a shorter time and at a lower cost. RM4HEALTH aims to stimulate innovation in continuous monitoring in healthcare and sports.
Our research team will investigate and develop algorithms for especially HF decompensation detection using measurement data collected during clinical trials. The main contributions would circle around following project targets:
– BCG, PPG and ECG signal processing analytics
– Detection algorithms for HF decompensation and detection, especially serial BCG combined with readily outputted parameters (HR, HRV, RR, activity…)
Machine Learning for Systems Pharmacology
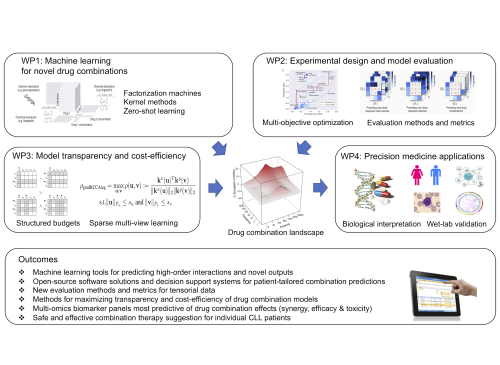
1.9.2021 – 31.8.2025
1000 000€ total, 330 000€ University of Turku
In the project we develop new machine learning methods for predicting the effects of drug combination treatments in complex diseases such as cancer, and implement novel clinical decision support tools that guide treatment decisions to optimize combinatorial therapies. This is expected to lead to increased treatment efficacy, individualization of therapy and reduced drug use and side effects, as well as reduced use of ineffective drugs and lower financial stress that the health care providers and the patients currently experience. The proposal is based on the top-level international expertise of the consortium on machine learning, systems pharmacology, tensorial data, and translational applications.
AI technologies for interaction prediction in biomedicine
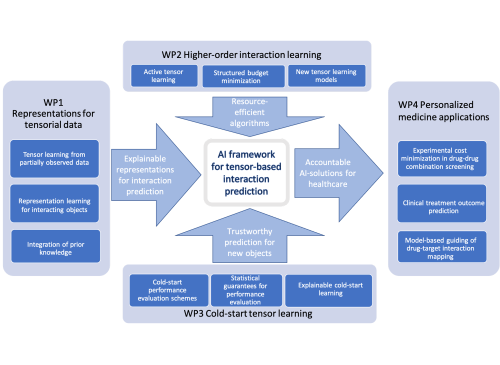
1.1.2022 – 31.12.2024
950 000€ total, 400 000€ University of Turku
In the project we develop new techniques and tools for AI tasks where the interaction of two or more objects is to be predicted. Such problems are prevalent in society, found in numerous applications, such as various link prediction problems in social networks, recommendation systems, among others. In the biomedical domain, prediction of binding of drug molecules to their molecular targets and responses of a patient to a combination of drugs are crucial interaction prediction tasks for enabling personalized and precision medicine.
The project advances state of the art in AI by tackling several key challenges related to interaction prediction with tensor methods: (i) learning new representations for structured objects to increase accuracy and explainability, (ii) developing methods for partially-observed fragmented datasets, (iii) increasing explainability of predictions through integrating symbolic prior knowledge in learning, (iv) data-efficient learning of models through active learning and structured budgets, as well as (v) new methods for trustworthy performance evaluation especially in cold start scenarios, where interactions of previously unseen objects need to be predicted.
Accelerate innovation in medical devices by “Moore for Medical”
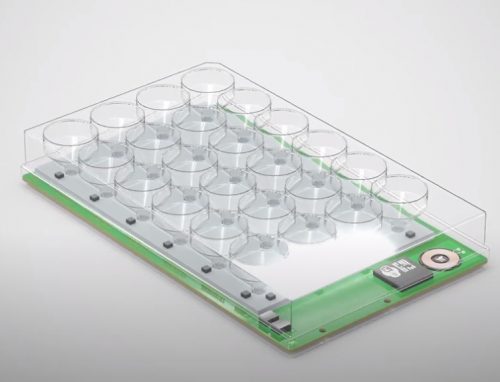
01/06/2020 – 31/05/2023
€66M total, €1.7M University of Turku
Moore4Medical will accelerate innovation in electronic medical devices. The project addresses emerging applications and technologies that offer exciting new opportunities for the Electronic Components and Systems (ECS) industry. Moore4Medical will focus on the development of open and enabling technology platforms for these emerging fields to help them bridge “the Valley of Death” in shorter time and at lower cost. With value and IP moving from the technology level towards applications and solutions, defragmentation and open technology platforms will be key in acquiring and maintaining European leadership in the forefront of affordable healthcare.
In Moore4Medical, a new cost-effective and user-friendly platform for long-term at-home monitoring and follow-up of users will be developed. The platform will feature artificial
intelligence and machine-learning-based algorithms. The developed solutions will be clinically tested and validated during the Moore4Medical project, and be thus ready for commercial
exploitation in late 2023. Moore4Medical approaches Remote Cardiac Monitoring (RCM) via two complementary approaches: (a) measuring the motion of the bed upon which the person is resting and (b) measuring chest motion via remote sensing.
Privacy-preserving AI for Synthetic and Anonymous Health Data
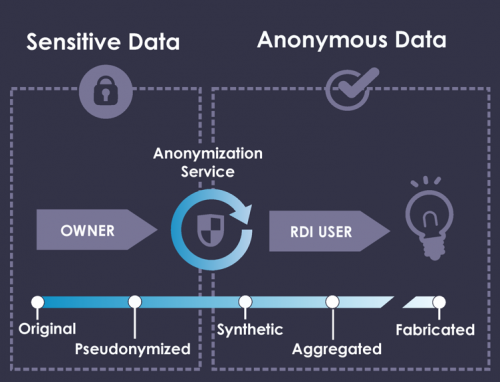
01/02/2020 – 31/08/2020 (Co-creation)
01/01/2021 – 31/12/2023 (Co-innovation)
€1M (Co-innovation)
100 000 € (Co-creation)
PRIVASA boosts data-driven research, development and innovation by making it easier to access and share health data without compromising individuals’ privacy. The core aim of the project is to add flexibility in the way that health data can be utilized in various stages of product development. This aim extends beyond technical solutions, as it also requires trust-building between owners, holders and users of the data. Transparency, legal aspects and ethics are the cross-cutting themes to be explored throughout the project.
PRIVASA develops tools that apply latest AI methods to support the growth of health technology businesses. Privacy-preserving algorithms convert sensitive data into a safe but useful anonymous format that is suitable for medical research, testing and validation. The use of anonymous data simplifies and speeds up the development cycles. The project addresses the special challenges related to medical images and free form patient records. PRIVASA offers explainable AI solutions that can be implemented as database interfaces or stand-alone services.
Monitoring solution for accessing lung and heart diseases
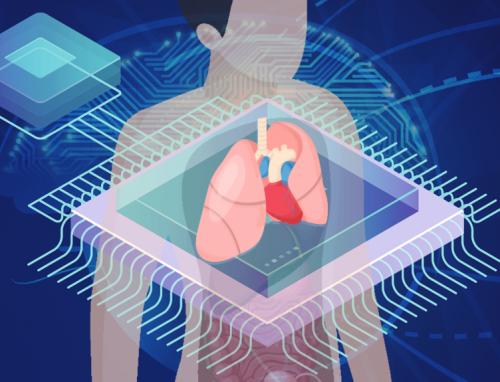
1.9.2020 – 31.8.2022
799 000 euros
The commercialization of a solution related to a heart failure (HF) and chronic obstructive pulmonary disease (COPD) is investigated in this project. This project addresses the problem that currently many of these patients are not able to recognize when their condition gets weaker. A solution enabling patients to monitor the progression of their disease and detect when the disease gets worse in time, thereby avoiding the acute hospital readmissions is investigated. The aim is to develop a proof-of-concept solution during the project.
Site of Research: Department of Future Technologies, University of Turku
Advanced packaging for photonics, optics and electronics for low cost manufacturing in Europe
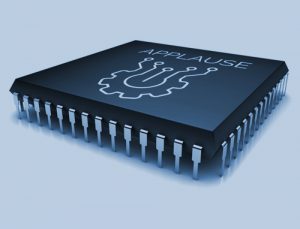
36 months, 2019-2022
€34M total, €1M University of Turku
A consortium of 31 European electronics packaging, optics and photonics key players, leading equipment suppliers and testing experts launched a new project, “Advanced packaging for photonics, optics and electronics for low cost manufacturing in Europe,” simply called APPLAUSE. The project fosters the European semiconductor value chain by building new tools, methods and processes for high volume manufacturing. The €34M total budget for the three-year project (2019-2022) is co-funded by Horizon 2020 and national funding agencies and industries, as a part of the Electronics Components and Systems for European Leadership Joint Undertaking (ECSEL JU). In Finland, national public funding is provided by Business Finland.
The six use cases of APPLAUSE are: (i) a substantially smaller 3D integrated ambient light sensor for mobile and wearable applications; (ii) a high performance, low cost, uncooled thermal IR sensor for automotive and surveillance applications; (iii) high speed datacom transceivers with reduced manufacturing costs; (iv) a flexible cardiac monitoring patch; (v) miniaturized cardiac implants with advanced monitoring capabilities; and (vi) an optical water measurement module with cost-effective packaging of components. With a budget of €1M, the UTU Health Technology Group participates in the flexible cardiac monitoring patch use case, where it is responsible for gathering and analyzing patient data and creating new advanced algorithms for detection of cardiac anomalies – atrial fibrillation, heart failure and coronary artery disease.
Project website: https://applause-ecsel.eu
Supporting Lifestyle Change in Obese Pregnant Mothers through Wearable Internet-of-Things
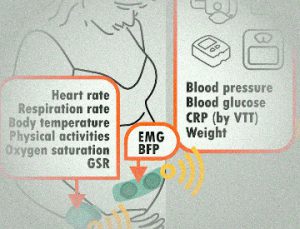
2018 – 2022
600 000 euros
Overweight (BMI > 25) and obesity (BMI > 30) are constantly growing problems causing many health consequences. Correspondingly, the prevalence of overweight and obesity in pregnant women is increasing. Pregnant women with obesity have indisputably increased risk for gestational diabetes mellitus, depression, and miscarriage, just to mention few. These pregnancy complications have negative effects on their unborn children. Physiological parameters and the information on pregnant women’s lifestyle and behavioral patterns –e.g., communication, eating, and recreation– can be used to investigate multifactorial processes that maintain obesity and leads to negative pregnancy outcomes. Moreover, monitoring of such parameters and patterns would help healthcare professionals and women themselves to monitor and tailor personalized interventions to minimize weight gain and adopt a healthier lifestyle during pregnancy. We believe the Internet of Things (IoT) technologies can be exploited to bring intelligence and ubiquitous monitoring to maternity care. In this project, an Internet-of-Things-based intelligent monitoring system is developed to detect and predict obesity-related pregnancy complications as early as possible. The cybernetic health concept is utilized by intertwining lifestyle and environmental data together with bio-signals associated with medical knowledge to develop a closed-loop system to make maternity care more effective, dynamic, and end-user driven. This is done via a platform that leverages portable devices and inexpensive wearable sensors, coupled with a multimodal event modeling, activity recognition, and life-logging engine. This research delivers a ubiquitous pregnancy monitoring service to end-users, mothers, and healthcare providers, enabling pregnancy events detection, prediction, assessment, and prevention.
Site of Research:
Department of Future Technologies, University of Turku (FT-UTU)
Department of Nursing Science, University of Turku (NS-UTU)
Turku University Hospital(TYKS)
Approximate Computing for Power and Energy Optimisation
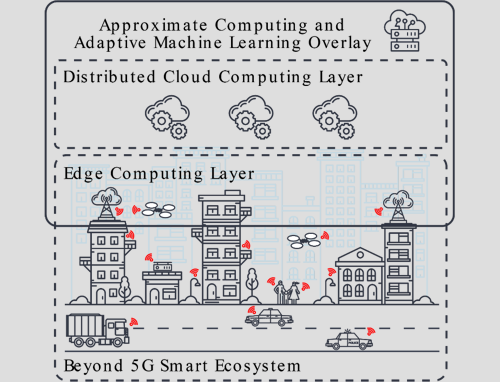
1.11.2020 – 31.10.2024
300 000 euros
15 Early Stage Researchers to tackle the challenges of future embedded and high-performance computing energy efficiency by using disruptive methodologies. Following the current trend, by 2040 computers will need more electricity than the world energy resources can generate. On the communications side, energy consumption in mobile broadband networks is comparable to datacenters. To make things worse, Internet-of-Things will soon connect 20 to 50 billion devices through wireless networks to the cloud. APROPOS aims at decreasing energy consumption in both distributed computing and communications for cloud-based cyber-physical systems. We propose adaptive Approximate Computing to optimize energy-accuracy trade-offs. Luckily, in many parts of the global data acquisition, transfer, computation, and storage systems there exists the possibility to trade off accuracy to either less power or less time consumed – or both. As examples, numerous sensors are measuring noisy or inexact inputs; the algorithms processing the acquired signals can be stochastic; the applications using the data may be satisfied with an “acceptable” accuracy instead of exact and absolutely correct results; the system may be resilient against occasional errors; and a coarse classification or finding the most probable matches may be enough for a data mining system. By introducing a new dimension, accuracy, to the design optimization, the energy efficiency can even be improved by a factor of 10x-50x. We will train the spearheads of the future generation to cope with the technologies, methodologies, and tools for successfully applying Approximate Computing to power and energy saving. The training, in this first ever ITN addressing approximate computing, is to a large extent done by researching energy-accuracy trade-offs on circuit, architecture, software, and system-level solutions, bringing together world leading experts from European organizations. In addition, we will provide network-wide and local trainings on the substance and on the complementary skills needed in both industrial and academic work life.