Supporting Lifestyle Change in Obese Pregnant Mothers through Wearable Internet-of-Things
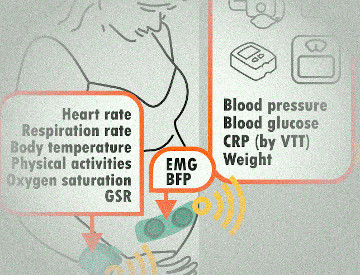
2018 – 2022
600 000 euros
Overweight (BMI > 25) and obesity (BMI > 30) are constantly growing problems causing many health consequences. Correspondingly, the prevalence of overweight and obesity in pregnant women is increasing. Pregnant women with obesity have indisputably increased risk for gestational diabetes mellitus, depression, and miscarriage, just to mention few. These pregnancy complications have negative effects on their unborn children. Physiological parameters and the information on pregnant women’s lifestyle and behavioral patterns –e.g., communication, eating, and recreation– can be used to investigate multifactorial processes that maintain obesity and leads to negative pregnancy outcomes. Moreover, monitoring of such parameters and patterns would help healthcare professionals and women themselves to monitor and tailor personalized interventions to minimize weight gain and adopt a healthier lifestyle during pregnancy. We believe the Internet of Things (IoT) technologies can be exploited to bring intelligence and ubiquitous monitoring to maternity care. In this project, an Internet-of-Things-based intelligent monitoring system is developed to detect and predict obesity-related pregnancy complications as early as possible. The cybernetic health concept is utilized by intertwining lifestyle and environmental data together with bio-signals associated with medical knowledge to develop a closed-loop system to make maternity care more effective, dynamic, and end-user driven. This is done via a platform that leverages portable devices and inexpensive wearable sensors, coupled with a multimodal event modeling, activity recognition, and life-logging engine. This research delivers a ubiquitous pregnancy monitoring service to end-users, mothers, and healthcare providers, enabling pregnancy events detection, prediction, assessment, and prevention.
Site of Research:
Department of Future Technologies, University of Turku (FT-UTU)
Department of Nursing Science, University of Turku (NS-UTU)
Turku University Hospital(TYKS)